Computer Vision:
Compared different Computer Vision libraries (YOLO3, Tiny-YOLO, OpenCV, Tensorflow) and their detection speed & accuracy on PC. Determined to use Tensorflow Lite for the highest frame rate.
1.YOLO3& Tiny-YOLO
You only look once (YOLO) is a state-of-the-art, real-time object detection system. Tiny-YOLO is a variation of the Yolo object detector. It is much faster than Yolo, yet less accurate. For reference, Redmon et al. report ~51-57% mAP for YOLO on the COCO benchmark dataset while Tiny-YOLO is only 23.7% mAP — less than half of the accuracy of its bigger brothers (Rosebrock, Adrian).We used a pre-trained model from https://pjreddie.com. Then we optimized tiny yolo by letting it only detects person and reduced input resolution from 416×416 to 200×200. However, it still only reaches 3.7 fps on MacOS. (We also tested out printing the mid-x position of the detected person, which is a value we will need for the robot to rotate and move accordingly).
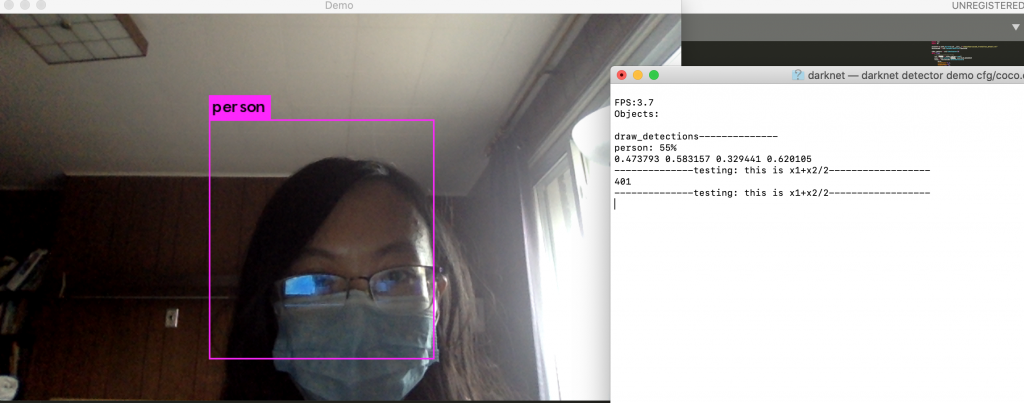
reference:
https://pjreddie.com/darknet/yolo/
https://medium.com/@madhawavidanapathirana/real-time-human-detection-in-computer-vision-part-2-c7eda27115c6
https://stackoverflow.com/questions/44674517/yolo-darknet-detecting-only-specific-class-like-person-cat-dog-etc
2.OpenCV
Haar Cascade Classifier is a popular algorithm for object detection. We used pre-trained Haar cascade models in OpenCV to detect human face in real time. It is the least accurate approach we’d inverstigated.
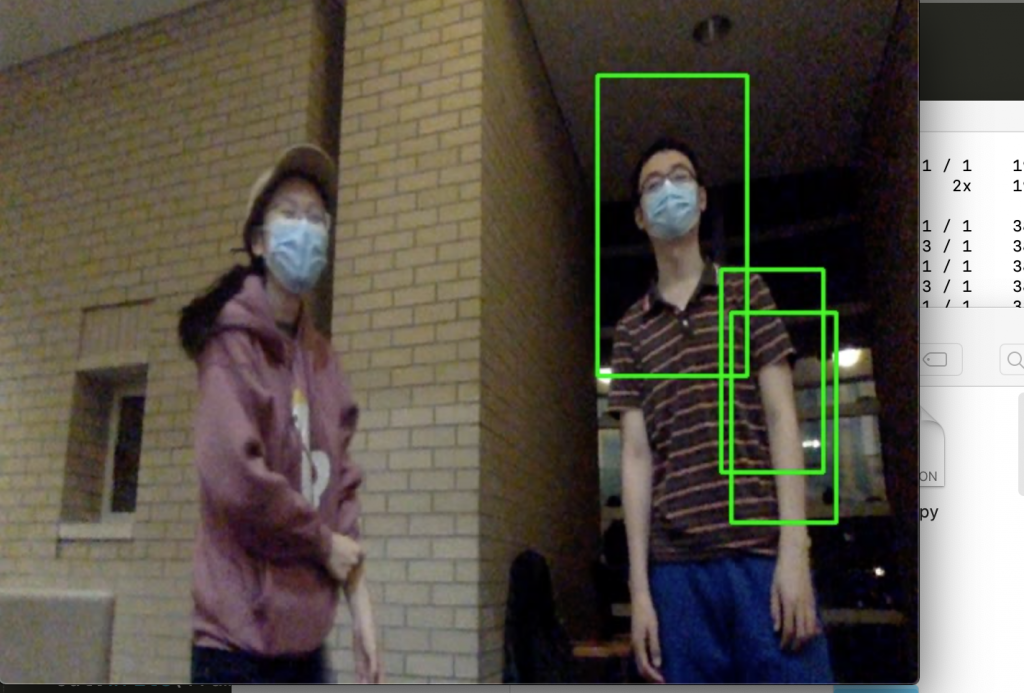
reference:
https://opencv-python-tutroals.readthedocs.io/en/latest/py_tutorials/py_objdetect/py_face_detection/py_face_detection.html
Real-time Face Recognition with Python & OpenCV
3.Tensorflow
TensorFlow™ is an open-source API from Google, which is widely used for solving machine learning tasks that involve Deep Neural Networks. We used Tensorflow Detection Model Zoo as our pre-trained model. It reached about 5-10 fps and its accuracy was good enough. Later, we found out that Tensorflow Lite is a even better optimized approach on raspberry pi.
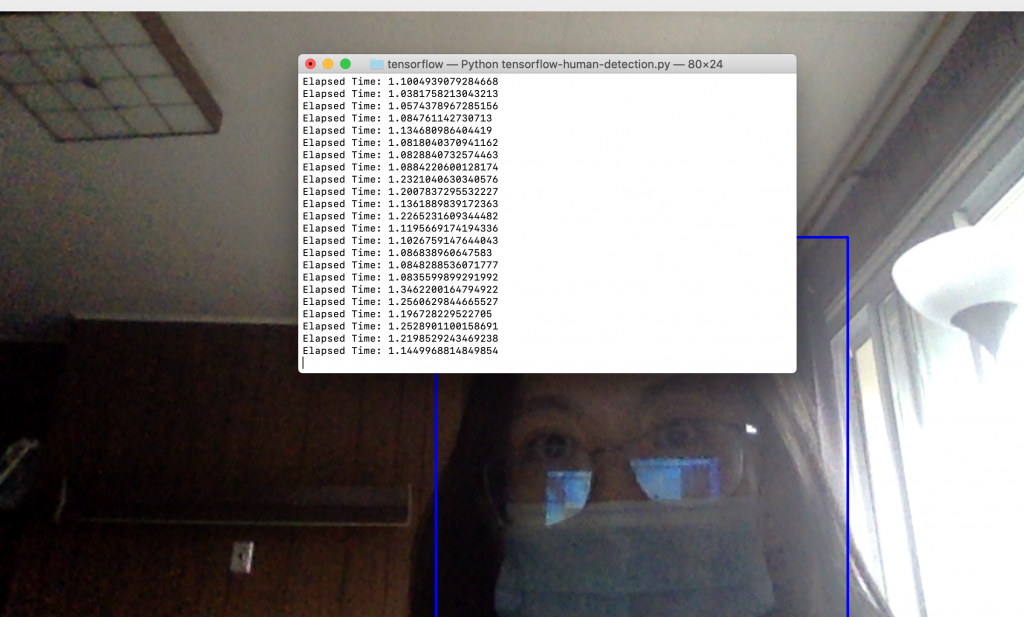
reference:
https://medium.com/@madhawavidanapathirana/real-time-human-detection-in-computer-vision-part-2-c7eda27115c6
https://github.com/EdjeElectronics/TensorFlow-Lite-Object-Detection-on-Android-and-Raspberry-Pi/blob/master/Raspberry_Pi_Guide.md
Hardware:
Create2 robotic base, raspberry pi, NCS2 and the Pi camera were all received on Thursday, 30th Sep. We set up the Pi environment (installing the OS and relevant libraries) and run the CV algorithm on the Pi with low FPS.
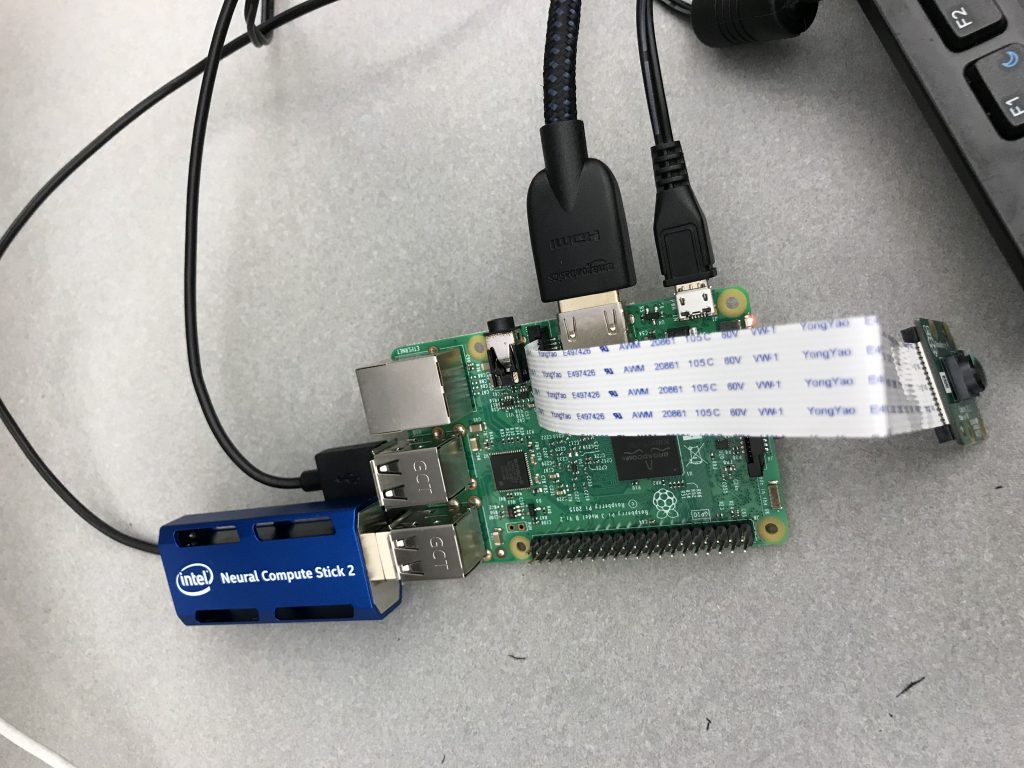
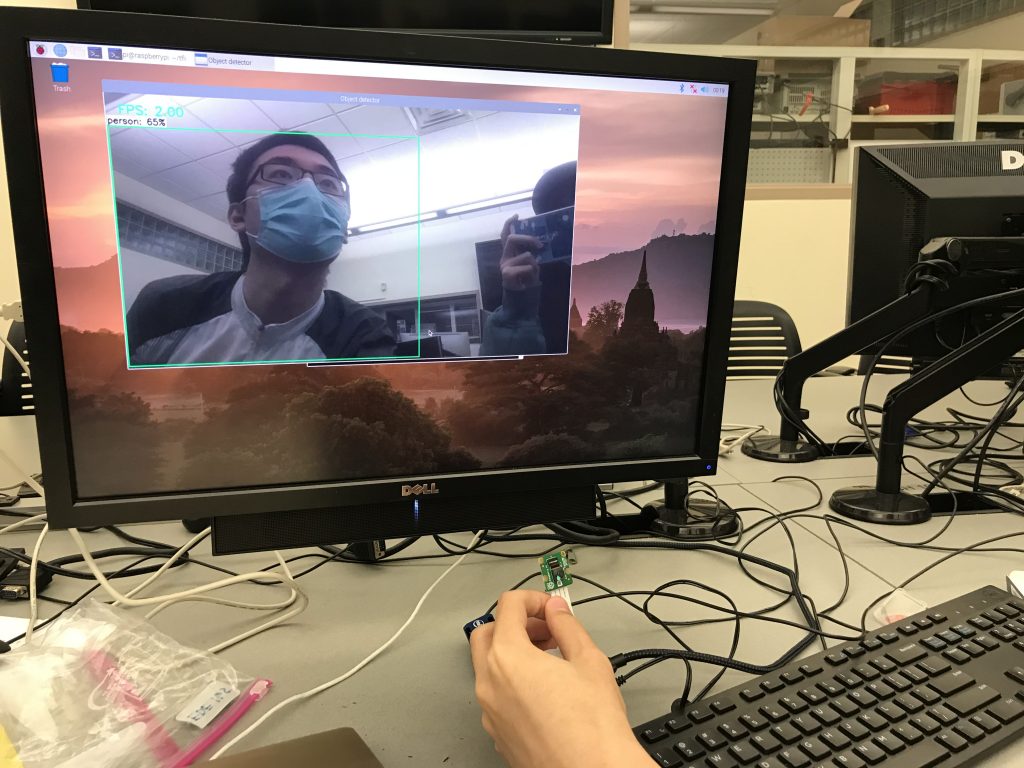
Difficulties
The Pi we borrowed from the ECE inventory is a Raspberry Pi 3 instead of Raspberry Pi 4, and there’s a huge performance difference between the two. Also, the NCS2 we chose as CV module is a relatively outdated piece of hardware, and is very hard to set up to coordinate with our choice of CV algorithm, Tensorflow Lite. After researching, in order to run the library, we need to use the NCS SDK and convert the module into NCS2 compatible, then run it on NCS2. On top of which, we found a result demo on YouTube and the result the video uploader was able to achieve on his Pi3 + NCS2 platform was 9-10 fps, which barely reaches the FPS requirement we had in mind.
Intended Solutions
We found another platform that outperforms the NCS2 and is specially optimized for running Tensorflow Lite. It’s called Google Coral, and it’s also a USB accelerator that can be connected to our Pi. The expected result of such hardware optimization will lead us to be running our CV algorithm at around 20 FPS. We also intend to get a refund for the NCS2 if possible.
reference:
OpenVINO, OpenCV, and Movidius NCS on the Raspberry Pi