For this week, I mainly worked on improving my text detection. After Monday’s interim demo with the professors, I realized that lots of important features are missing in my part for demo so I spent next few days to improve the flaws. Though it is not complete, I added a temporary dictionary that contains names of products and its descriptions. For instance, the main difference between Advil and Tylenol is the substances. Advil contains ibuprofen and Tylenol contains acetaminophen. Because I may not always extract the name of brand, I added some distinct words that is specific to the product so that it can recognize the product even if the brand name is not recognized. I also added a list of words that extracted from the image after text preprocessing has been done.
I also worked on correcting the text orientation. For testing purpose, I am testing with ideally positioned images now, but I know the user won’t always place the object in an ideal position. The text may be upside down or rotated a bit depending how the user places it so I used Tesseract’s image_to_osd function to check whether the text is correctly positioned. However, I am getting an error that says the resolution of image is 0 dpi and I could not figure this out yet so I think I am going to write a code on my own to rotate the images if text has not detected in the original image.
I worked on logo detection a little as well. As the professor said, this isn’t my priority yet, but I tested around some of the code. I trained a model using AlexNet and saved the best model as .pt file, but I haven’t tested with my real-world image yet.
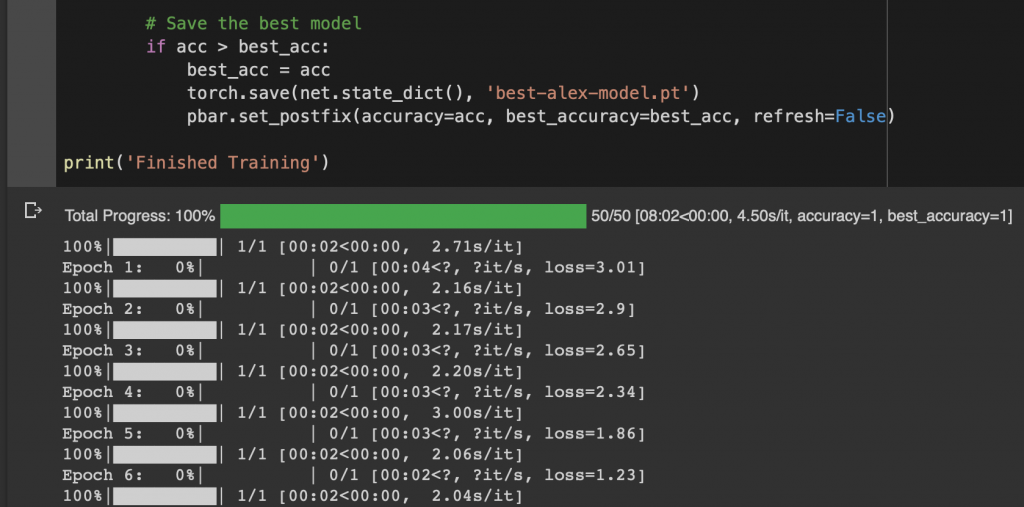
For next week, I am hoping to improve the efficiency of my code. Because I am testing around within my code, the efficiency is not so great. The current code extracts text from the original image, grayscale image, blur image, and both grayscale and blur image, and then match the product with all of those texts. It takes up to ~18 seconds depending on the size of the image and amount of text in the image. For example, when I run my code with an image of front of Advil, it take 2.5 sec to run everything. However, with an image of back of Advil, it takes 17.4 sec to run everything and get the result. The main difference is the inconsistency of image size I am using for the testing and amount of text in the image. I am planning to stop my code once a product is matched.
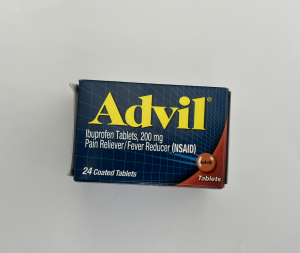
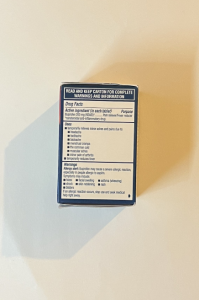
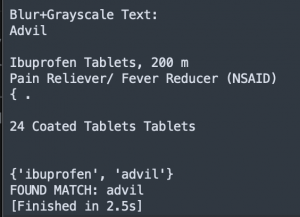
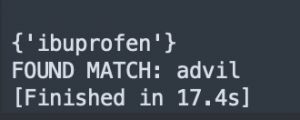
Frankly, I think I am still a bit behind on the schedule, but once I get the text orientation and efficiency figured out, I think I am pretty good on my own schedule.