Find our demo video here!
Team’s Status Report for April 30
What are the most significant risks that could jeopardize the success of the project? How are these risks being managed? What contingency plans are ready?
Our most significant risk is not putting adequate time into the presentation of our project. It is easy to spend all our time making marginal improvements on the device, but at this point, our system is mostly finished. The poster, demo, and final report are substantial amounts of work. It is important that we begin working on these assignments in parallel with our current testing and adjustment.
Were any changes made to the existing design of the system (requirements, block diagram, system spec, etc)? Why was this change necessary, what costs does the change incur, and how will these costs be mitigated going forward
After the final presentation, Professor Savvides advised us that one camera would be sufficient for our proof of concept and that we shouldn’t waste resources adding the second camera. This update will save us time and money down the stretch.
Provide an updated schedule if changes have occurred.
- Sunday-Tuesday: Poster + Test
- Wednesday-Saturday: Report + Demo
Justin’s Status Report for April 30
What did you personally accomplish this week on the project? Give files or photos that demonstrate your progress.
On Sunday I worked most of the day preparing for the final presentation. On top of working on the actual presentation, I finished the neural network for photo editing. This involved creating the final dataset for the photo editing, training the model, and testing with people outside of the project. During the week, I attended the final presentations in class. I also worked on resolving an installation issue on the Jetson Nano. Our current versions of PyTorch and CUDA were incompatible.
Is your progress on schedule or behind? If you are behind, what actions will be taken to catch up to the project schedule?
I am on schedule with the most recent team schedule.
What deliverables do you hope to complete in the next week?
Demo : )
Sids Status Report for April 30th
What did you personally accomplish this week on the project?
This week I focussed most of my work on working on finishing the integration of the system and beginning to procedures needed for testing.
On the integration side, we are debugging the PyTorch and Cuda versions on the Jetson since there was some compatibility issue that arose when we tried running the script that had the detection integrated with the camera simple search. Justin is currently taking a look at this and as soon as that is done we move on to testing the system implementation with our one camera approach we had discussed in the last meeting with Professor Savvides.
On the testing end, I looked through the WCS Camera Traps Dataset once more and this time looked for images that were not in our dataset and had pictures of different animals in a variety of orientations (all photographed well).
Some of these are attached below:
Once I finish printing out the pictures I can get the cut-outs and find a way to mount and place them so we can place them around the setup for testing. To test out different lighting conditions, I found a place we can use where we can control the light exposure and hence test at different distances and in different light conditions that simulate the real environment.
Is your progress on schedule or behind? If you are behind, what actions will be taken to catch up to the project schedule?
My progress on the project is a little behind because I was unwell this last week. In the meantime, I made progress with Integration and Testing as described above and to get back on schedule I am going to focus all my effort on finishing the project in the coming few days. Once we finish debugging, I can spend time on testing and refining the system as needed which should be something that can be accomplished with repeated testing and tweaking of the system.
What deliverables do you hope to complete in the next week?
Finish testing and refining the system as much as we can in order to have a smooth and well-functioning demo
In the meantime, I will finish the deliverables as needed i.e. the poster, report and video.
Sids Status Report for April 23rd
What did you personally accomplish this week on the project?
The majority of my work this week involved finishing the process of training the detection model properly and ensuring that this was done properly.
I faced many problems with this process; first, our setup on Colab did not work in time either due to high latency during the scanning of the dataset files from google drive. Following this, I decided to use my teammate’s old desktop for training the model since it had a GPU. After managing to transfer all the files to the desktop using the public sharing URL provided by google drive.
I set up the environment needed for training the neural net on the desktop and this took much longer than I had expected. We ran into many problems and with my team’s help, I was able to modify the Python and CUDA versions on the desktop to be compatible with each other and YOLOv5. We also had to make changes to the CUDA and PyTorch versions that were running on the JESON Nano based on the changes we made.
While the model was training, we worked on the search algorithm for the camera on the JETSON. Once the model was trained we got statistics regarding the recall and accuracy which we were happy with.
Recall ~ 93%
Acuraccy ~ 92.8%
After adding code to the scripts on the JETSON, the detection model can be integrated into the algorithm. We move the trained best weights and call the predict function for every frame the camera sees until the detection model tells us to transfer control to track.
We planned out the testing process and I picked images we are going to print to test the project in the coming days.
Is your progress on schedule or behind? If you are behind, what actions will be taken to catch up to the project schedule?
The progress is slightly behind due to all the problems faced while training the detection model and the fact that errors would show up hours into running the training script.
To make up for this, my team helped me with implementing a basic simple search algorithm with the camera for now and we plan to speed up our plans for testing which would put us back on track.
What deliverables do you hope to complete in the next week?
Test results for the detection and integrated system.
Team’s Status Report for April 23
What are the most significant risks that could jeopardize the success of the project? How are these risks being managed? What contingency plans are ready?
We are finishing integration this weekend and working through testing. At this point, our biggest risk is not being able to upgrade our system to two cameras. Because the cameras are not in the same location, an additional alignment code will be necessary. This may be difficult to get operational. As a contingency plan, we can stack the two cameras together. One can be permanently zoomed out (for tracking) and the other can zoom in (for photographing). This approach is simpler and would not be much less effective.
Were any changes made to the existing design of the system (requirements, block diagram, system spec, etc)? Why was this change necessary, what costs does the change incur, and how will these costs be mitigated going forward
After speaking with one of Professor Savvides’ Ph.D. students, we realized that using two MIPI-CSI cameras on our version of the Jetson Nano is not possible even with our multi-camera adapter. This is because the camera stream must be terminated before another camera can be accessed. We found that the best way to troubleshoot these problems is by purchasing a USB IMX219 camera. This is the same model we currently have, so our code will be compatible, but the USB input can be streamed simultaneously to the MIPI-CSI. The camera will fit on our current pan tilt system and is only $35 on amazon. We will cover this expense ourselves and can get two-day shipping.
Provide an updated schedule if changes have occurred.
We will add our second camera this week after our initial round of testing. This will go under the ‘modifications’ time previously allocated.
Justin’s Status Report for April 23
What did you personally accomplish this week on the project? Give files or photos that demonstrate your progress.
With deadlines upcoming, I contributed to multiple areas of the project. The most crucial area I worked in was implementing the search algorithm on the robotic system.
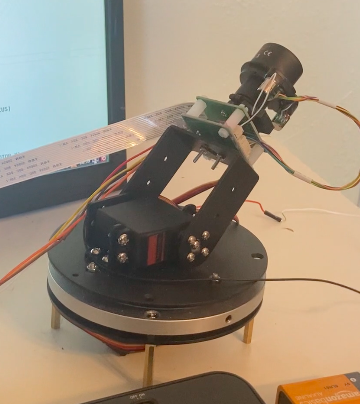
The second thing I worked on was helping troubleshoot the training of our detection CNN. We tried training this model on a laptop and on google Colab, but neither of these approaches were feasible. So instead we used my desktop. This ended up being more difficult than anticipated due to incompatibility between our CUDA and Pytorch versions. Afterward, I worked with my group members to install PyTorch on the Jetson Nano to use the detection model.
Finally, I continued to make progress on the photo editing algorithm. Our goal is to have a CNN which outputs amounts to apply various image algorithms. To generate data for this training, we need the algorithms to be reversible to determine the target editing modification. Some inverse algorithms (ex. tint inverse) could be mathematically derived. Others (like sharpening and blurring) required experimentally finding a relationship that minimized reconstruction error.
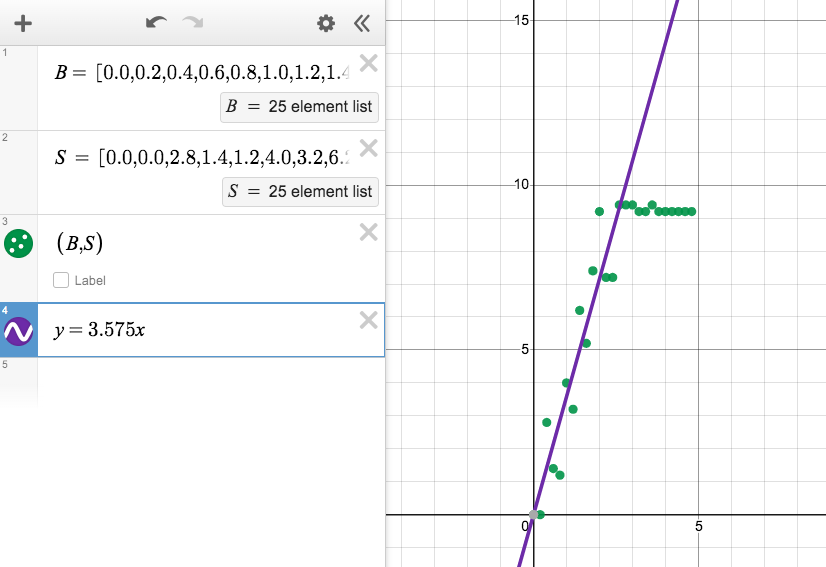
Is your progress on schedule or behind? If you are behind, what actions will be taken to catch up to the project schedule?
I am on schedule with the most recent team schedule.
What deliverables do you hope to complete in the next week?
Complete test results and modifications for the system.
Team’s Status Report for April 16
What are the most significant risks that could jeopardize the success of the project? How are these risks being managed? What contingency plans are ready?
At this point, we have two major risks. The first risk is that multiple pieces (detection, tracking, hardware, editing) of our system fail to meet the quantitative requirements. If this is the case, we will not be able to team up to make final system revisions. We would have the capability to work separately on adjustments, as this is how we began work this semester. However, this would not be the ideal situation and could possibly complicate a second integration round.
The second major risk is adding a second camera to our system. This process will hopefully go smoothly, but with the difficulty we faced getting the single-stream working, there is a possibility that adding a second camera could be equally challenging. If it becomes too difficult to integrate the second camera, then we should focus on getting the best possible single-camera system. Our goal is to have a strong and functioning proof of concept, and while not ideal, this can be accomplished with one camera.
Were any changes made to the existing design of the system (requirements, block diagram, system spec, etc)? Why was this change necessary, what costs does the change incur, and how will these costs be mitigated going forward
No changes were made to the system.
Provide an updated schedule if changes have occurred.
No schedule changes.
Justin’s Status Report for April 16
What did you personally accomplish this week on the project? Give files or photos that demonstrate your progress.
One of our largest integration problems has been streaming the camera’s video using python 3. The demo code/library for the Pan Tilt Zoom camera was written for python 2 and throws an error when run in python 3. This is a problem because all the code we have written is in python 3. I had previously tried fixing the code by changing the streaming method to write to a pipe or using ffmpeg. After working through the errors in these methods, I realized that the problem was actually with the Threading library. I had been confused by the generic error message combined with a nonfatal error for the GStreamer library which occurred directly before (which I thought was the problem). I have spent the latter half of the week rewriting the JetsonCamera.py file and Camera class to avoid using this library, while still interfacing in the same way.
Whenever I was away from my house and did not have access to the Jetson, I continued working on the photo editing feature. I am working on training a photo editing CNN to automatically apply different image editing algorithms to photos. I plan to use the Kaggle Animal Image Dataset because it has a wide variety of animals and also has only well-edited photos. I am still formatting the data, but I do not anticipate this task should take too long. This part is not a priority, as we identified it as a stretch goal.
Is your progress on schedule or behind? If you are behind, what actions will be taken to catch up to the project schedule?
I am on schedule with the most recent team schedule. I plan to finish integration tomorrow to present in our meeting Monday.
What deliverables do you hope to complete in the next week?
A full robotic system that we can test with.
Sidhant’s Status Report for April 16
What did you personally accomplish this week on the project? Give files or photos that demonstrate your progress.
I made some major changes to the detection algorithm this week. I was able to understand the error with the bounding boxes which was a major step in the right direction.
While fixing this, I came across some problems related to the naming of files (because of Linux and macOS differences). Attempting to fix the naming of files should have been trivial but did not work as expected; I tried seeking help with the issue but decided on creating a script that renamed the downloaded dataset files to avoid any errors in the future.
I re-partitioned the dataset correctly and edited the representation of JSON files and the annotations for the dataset.
Following this, I was able to test the edited bounding boxes. After running tests for sets of random files (from the train, validation, and test data) we saw good results and after some scaling, I was satisfied with the way they were represented (according to the YOLOv5 format).
After testing, I was able to attempt re-training the model. I specified the correct destinations and ensured that all hyperparameters defined in the hyp.scratch.yaml and the train.py files seemed correct. Since training is a long procedure, I consulted my team for their approval and advice and then moved on to running the training script for the neural net.
The issue I came across was that training on my laptop was seeming to take very long (~300 hours) as shown below.
I asked Professor Savvides for his advice and decided to move training to Google Colab since this would be much quicker and wouldn’t occupy my local resources.
All datasets and YOLOv5 are being uploaded to my Google Drive (since it links to Colab directly) after which I will finish training the detection neural net.
In the meantime, I am refining the procedures outlines for testing and setting a timeline to ensure adequate testing by the end of the weekend. While doing so we came across some problems with relaying the video of the physical setup through the JetsonNano. After some research, I am now focussing on implementing one camera until we can get some questions answered regarding using both in real-time.
Is your progress on schedule or behind? If you are behind, what actions will be taken to catch up to the project schedule?
We fell behind a little bit due to roadblocks related to the setup, real-time video feed, and training the neural net correctly.
By the week done this week, I should be back on schedule if I am able to finish training and test the algorithm to some extent. We still need to get the physical setup to communicate with the Jetson exactly as we desire and as soon as this is done it should be a simple procedure to integrate this with the tested detection algorithm.
What deliverables do you hope to complete in the next week?
By the end of next week, I will have the detection algorithm finished and tested as described above. Further, I expect to be able to take a scan of a room using the setup as I would to detect animals, and following this, I hope to integrate and test the features of the project.
This would mean we can focus on polishing and refining the robot as needed as well as fine-tune the different elements of the project so they work well together.