Progress
This week, I kept working on getting the point to work with a 3×5 bin larger room. With the new dataset, the multitask model was getting 0.94 validation x accuracy and 0.95 validation y accuracy, but the results were not smooth when running it on a test video and panning the point around the room.
So, I tried using a regression output rather than a categorical output, such that the model would be able to relate information about how close the bins are instead of treating the bins as independent. This gave a much smoother result, but still has issues in the panning video.
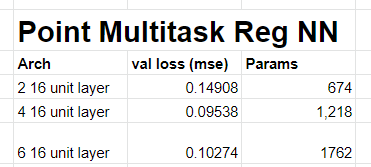
To address the smoothness in the testing videos, I added additional data of moving my arm around while pointing to a bin. This adds noise to the data and lets the model learn that even though I am moving my arm, I am still pointing to a bin. This worked well when I tried it on pointing to one side of the room. I will continue to try this method to cover more parts of the room.
I currently am manually evaluating the test videos, if I have time I will try to add labels to it so I can quantatitively test it.
Deliverables next week
Next week, I will have gotten the point to work on the entire room.
Schedule
On schedule.