In the previous week, I combined the 3 different computer vision algorithms into one program that invokes all of them. Along the way, I found out the blob detection was not as effective as I thought under dim lighting conditions. As a result, I had to make modifications to the blob detection by changing several parameters regarding eroding excess lines.
You may have noticed the image is a result of an object recognition and not of an image cassification network. There are several reasons for this. One, the dataset we collected had multiple types of meat strewn together, and at that moment, our team realized that if someone placed multiple types of meat on a plate in front of the robotic arm, a classification system is not robust enough to know that and may cause undercookng of meat. Another reason is that if the blob detection fails to work in a way we want, object recognition algorithm is our backup mitigation technique. While object recognition is slower than blob detection, it’s still sufficiently fast enough for our desired metrics.
What you see below is the result of YOLOv5 trained on 150 epochs on a tiny data set (only 20 images) augmented to be 60 images in total. The batch size each epoch was 12 images each. YOLOv5 was selected due to its speed advantage and its active community support online.
Currently on track to complete by the completion date indicated on the schedule, which is Monday, at least I am mostly done. The hesitation is because the integration period will provide a chance to add to the dataset, and that would require more training on the network.
By next week, I hope to begin the integration of the subsystems by having the files uploaded onto the Xavier. Hopefully that would lead to an improvement in detection time also.
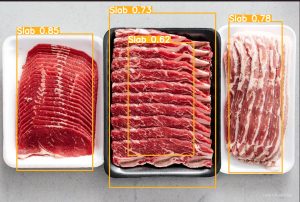