Team
With the upcoming design presentation, we knew we had to make some important decisions. We’ve decided to use PoseNet and create a web application, which are two major changes from our original proposal. This is because we discovered that our original design, which was using OpenPose in a mobile application, would run very slowly. However, this change will not affect the overall schedule/timeline, as it is more of a lateral movement than a setback. Our decision to abandon the mobile platform could jeopardize our project; to adjust, we decided to offload processing to a GPU, which will make our project faster than it would have been on mobile.
Kristina
This week, I worked with Brian and Umang to test the limits of PoseNet so we could decide which joint detection model to use. I also started creating the base of our web application (just a simple Hello World application for now to build off of). I haven’t done any web development in a while, so creating the incredibly basic application was also a good way to review my rusty skills. Part of this was also trying to integrate PoseNet into the application, but I ran into installation issues (again…like last week. Isn’t set up like the worst part of any project) so I ended up just spending a lot of time trying to get TensorFlow.js and PoseNet on my computer. Also since this upcoming week is going to be a bit busier for me, I made a really simple, first-draft sketch of a UI design to start from. For this next week, my goals are to refine the design, create a simple application we can use to start gathering the “expert” data we need, and to start collecting the data.
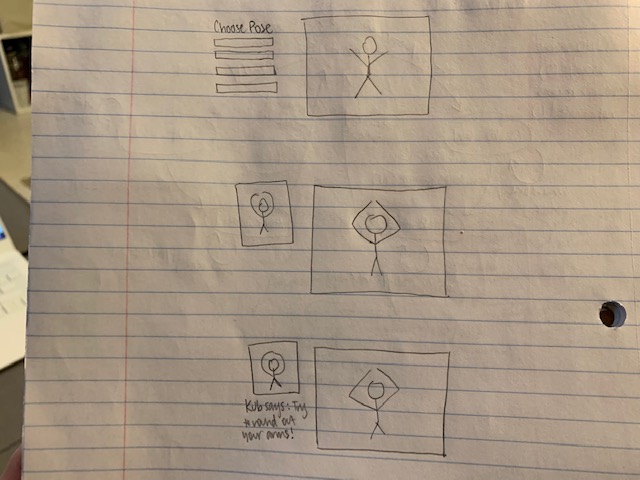
Brian
This week I attempted to find the mobile version of openpose and have it run on an iphone. Similarly to last week, I ran into some issues during installation, and decided that since we already had a web version running, it was better to solidify our plan to create a webapp and trash the mobile idea.
Afterwards, I decided to get a better feel for the joint detection platform, and play around with tuning some of the parameters to see which ones yielded the best accuracy. This was mainly done by manual observation of the real time detection as I tracked the movement of what I assumed were dancelike movements. I also took a look at the raw output of the algorithm, and started thinking about the frame matching algorithm that we would like to use to account for the difference in speed amongst the user and training data. I also worked on creating the design documents. For the next week, I would like to work more with the data, and see if I can get something that can detect the difference between joints in given frames.
Umang
This week I worked with Brian to explore the platform options for our application. We found that mobile versions will be all to slow (2-3 fps without an speed-up to the processing) for our use case. We then committed to making a web app instead. For the web version, we used a lite version of Google’s pretrained posenet (for real time estimation) to explore latency and estimation methods. With simple dance moves, I am able to get the estimate of twelve joints; however, when twirls, squats, or other scale/orientation variants are introduced, this light posenet variant loses estimates. As such, this coming week, I want to explore running the full posenet model on a prerecorded video. If we can do the pose estimation post hoc, then I can send the recorded video to an AWS instance with a gpu for quicker processing with the entire model and then send down the pose estimates.
I still need to work on the interpolation required to frame match the user’s video (or frame) with our collected ground truth. To evade this problem, we are going to work stills of Kristina to generate a distribution over the ground truth. We can then query into this distribution at inference time to see how far the user’s joint deviates from the mean. I hope to have the theory and some preliminary results of this distributional aggregation within the next two weeks.