Work Done
Due to the amount of time needed to tag positive and negative images for the Haar classifier (last week it took 4+ hours to tag ~60 images, and to train a better classifier I would probably want 200-300) I thought it would be more efficient to look into other, more accurate classifiers (pretrained) online. I found a few; the best performing one so far is one that uses the YOLOv4 object detection model. Below is a screenshot of how it performs on one clip of the Fifth and Craig intersection that Zina took last week:
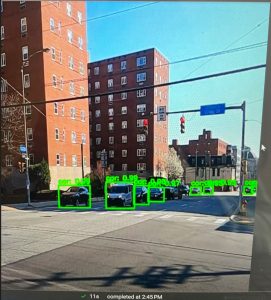
It is evidently fairly accurate; however, as expected, it does take quite a bit more time. After timing it, it takes 5-6 seconds for it to detect cars on a captured frame (but more than 10 if there are many cars. The above screenshot took 11 seconds.)
I’ll continue to remove unnecessary computations (initially the above screenshot took 15 seconds), but I’m looking into other models that can do faster computations, since this is simply too long for our project requirements.
We also ordered the new camera and it came yesterday; I will try to access the stream via RTSP today/tomorrow on my apartment WiFi, since the camera can only connect to the same WiFi that my phone is connected to (so I will work with one of my teammates on Monday to get the camera set up on their phone so that it can hopefully connect to my WiFi hotspot through that. I was unable to get it to connect to my phone’s hotspot while only using my phone.)
Schedule
We have the interim demo coming up, and I will have a faster object detection model ready by then. Due to uncertainties regarding the camera, I’m unsure if I will be able to have the model running on a live camera feed by then.
We also need to start integration, so Kaitlyn and I plan to work together next week to get the SUMO simulation set up on the RPi. I plan to get the object detection algorithm running on the RPi as well (which will be a bit of a task since as of now I’ve only gotten it running on Google Colab.)
Deliverables
By the end of next week, I will:
- Get the SUMO simulation running on the RPi
- Reduce the delay of the object detection model
- Attempt to access the video feed from the IP camera through RTSP