Kevin:
Update for week 4
- Accomplishment #1 description & result
- This week our first revision of PCBs arrived along with the parts needed to assemble it.
- We assembled the PCB using solder paste placed of the exposed pads. The solder paste was placed using a stencil cut from a precise vinyl cutter. The board then went into an oven where a specific pattern of heating is used to properly solder the components.
- Once out of the oven we fixed a few issues with the soldering, namely some bridges on the power ICs.
- Upon testing the PCB, we discovered the ESP32 was getting very hot, much hotter than it should be in normal operation. The issue was that 5V was being put into the 3.3V pin on the ESP32. I did not thoroughly read the datasheet for the ESP32 and thought 5V would not harm the device, this is incorrect. The boost converter used after the battery manager does does not regulate higher voltages down to 3.3V, instead it only boots up the 3.3V. This resulted in the 5V coming from the USB going into the processor without being stepped down.
- We can’t simply supply 3.3V on the power in line because the battery manager needs at more than this to operate and so the output voltage is only about 2V, this also won’t charge the battery.
- We decided to order another PCB with changes to supply the proper 3.3V needed. We will use a Buck-Boost converter which will step both up and down to regulate the voltage to the processor at 3.3V.
- I chose to use the TPS63001 chip for this task. It has voltage input range of 2.4V to 5.5V, which matches what we need quite well. The output is fixed to 3.3V with a ripple of about 10mV. The chip has a power saving mode, which we will be using, that lowers the switching frequency when possible.
- We also discovered one of the the ground pins on the ESP32 was not connected because the ground pour was slightly smaller than expected preventing a connection.
- We were able to see some data from the vesper mic vout pin since the breakout section was working properly.
First revision pre-assembly
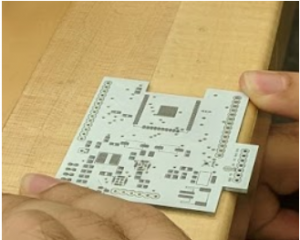
- Upcoming work #1 description & expectation
- Next week I will be working on finishing the schematic for the new board as well as routing. I will also order the new parts needed for this board and the board itself.
- I will also begin to research more on background noise reduction
- If the boards arrive on time we will try to assemble and test those as well.
Update for week 5
- Accomplishment #1 description & result
- This week I finished routing the new board, Rev 0.2. I kept the breakouts for the ESP32 and the vesper mic. I fixed the ground issue for the one pin the processor and of course added the new buck-boost converter and its peripherals.
- The board was ordered over spring break along with the new parts.
- While waiting for the board to arrive, I was able to research more removing background noise from our audio data. I have a matlab filter used for hearing aids I want to try as well as audacity’s noise reduction system. I need to collect noisy data to test these methods. Audacity is open source so the code for their noise reduction system is available in C++ which I may adapt to our needs if it works well. In preliminary tests it appears to work quite well. The user selects an area of the signal that is only background noise and the algorithm does its best to remove only similar sounds without destroying the good signal.
- The new boards and parts arrived by Wednesday and Ronit assembled it on Thursday morning. We discovered the output of the buck-boost converter was not regulating the output voltage to 3.3V. After careful inspection we found that the feedback pin had to be connected to the output voltage even on the fixed 3.3V version. I soldered a wire on the board between the to pins and it now works very well.
- The board can be powered from 5V USB or our LiPo battery without issue. At first glance it also appears to charge the battery correctly but more testing needs to be done. Importantly, the board connects to the computer and can be programmed.
- The Vesper mic appears to react to noise levels as well.
- We made a quick fix to the old board as well. We soldered a wire into the 3.3V line so we can power the board direction with 3.3V. We need to test if the battery can power it but we will not be able to charge due to the issues discussed in last week’s report.
Rev 0.2 Board, main changes are centered around part U2, the new buck-boost converter.
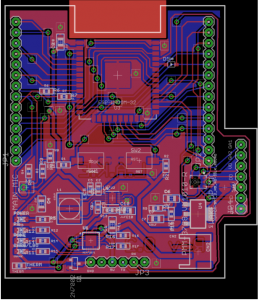
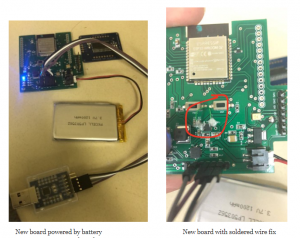
- Upcoming work #1 description & expectation
- I will be collecting noisy data and looking at the effectiveness of the background noise algorithms I researched this week.
- If the board bring up goes well, I will start designing out Rev 1.0 for the PCB which will be more stripped down and as small as possible.
James:
Update for week 4
- Accomplishment #1 description & result
- This week, I worked with Ronit to begin collecting labeled sample data to work on over the break. I began researching different features used and methods to extract them from papers which accomplished similar attacks.
- Past work has shown that cepstrum features do yield better classification accuracy than FFT. However, we want to test this for ourselves and compare FFT, cepstrum, and a concatenation of both.
- Upcoming work #1 description & expectation
- In the upcoming weeks, I will work on extracting different features from keystrokes in an automated manner and attempt to cluster different keys based on these features.
- I will also need to further explore the potential classification algorithms to use. During my research of which features to use, I found a comparison of different classification algorithms and their accuracy. Interestingly, a linear classification model yielded better accuracy on test data than both a neural network and a gaussian mixture model. I will try to compare both linear classification as well as the original strategy we planned on using, k-means.
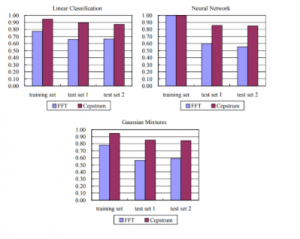
- Changes to schedule description
- No major changes have been made to the schedule. However, we have been shifting responsibilities around as needed in order to allow Kevin to fully complete the next revision of our PCB.
Update for week 5
- Accomplishment #1 description & result
- This week, I worked more on the feature extraction from the labeled keystroke data. I extracted both FFT and cepstrum features from the entire window of the push peak of the keystroke. I am currently ignoring the release peak as it is less intense in energy and sometimes difficult to pick up in the presence of background noise.
- Using the FFT and cepstrum features, I attempted to classify a few different letters using k-means. When classifying a combination of ‘q’, ‘a’, ‘k’ and the space bar, I found that both the ‘k’ and spacebar were clustered fairly accurately, yielding no more that 2-3 erroneous classifications out of 20. However, the letters ‘a’ and ‘q’ were misclustered into all four clusters
- I thus attempted to increase the feature set. I began by taking FFTs and cepstrum features over sliding windows of 5ms with a 3.75ms overlap. Unfortunately, this did not alleviate the issue.
- I also helped Kevin troubleshoot the newly arrived second revision of the PCB. Unfortunately, there was a missing connection on one of the inputs to the buck-boost converter, and we manually soldered on a wire to bridge the connection. This corrected the issue.
- Upcoming work #1 description & expectation
- I will further explore different options and features for classifying the keystrokes effectively. We are currently having some difficulty effectively clustering the data, and this is currently the biggest risk area in the project. I will take a closer look at the previous work done in this area and attempt to replicate their methods as described.
- We also hope that TDoA will serve as a highly distinguishing feature. Now that we have multiple working sensor packages (both breadboarded and PCB), we can begin to incorporate TDoA data into the clustering.
- Should we still find ourselves unable to find the correct features to cluster upon, we may need to explore different keyboards, typists, and typing styles.
- Changes to schedule description
- We are falling behind schedule in terms of machine learning and signal processing. Fortunately, the PCB design has been mostly finalized. This will allow us to fully shift our focus to these areas. Because of the ample time for testing and integration we alloted to ourselves, we should still be on track to complete the project.
Ronit:
Update for week 4
- Accomplishment description & result
- We collected data for each individual key. We recorded 30 keypresses pressed with the index finger.
- I worked with James to extract features from the sound. We got some features but decided to work on them further after spring break.
- I worked with James on keystroke separation, we read the paper Acoustic Keyboard Emanation Revisted Zhung et al. It described a means of extracting keystrokes from a sound clip by creating 10ms windows and examining the the change in the energies.
- Upcoming work description & expectation
- After spring break, we will probably have the new batch of PCBs.
- Once we have a working remote listening device, I will make the processor sleep and only wake up when sound is detected.
- Once we have a working sensor package, we will need to work on adapting our network stack to support data from two devices, so that we can extract time difference of arrival.
- Changes to schedule description
- I will be helping James with the machine learning from here onwards, we need to try and reach out MVP as soon as possible
Update for week 5
- Accomplishment description & result
- Kevin made changes to the PCB over spring break and ordered new ones.
- I assembled the PCB, there was some issues with the power management circuit, but we managed to solder a wire on and fix it.
- I was able to flash our program onto the PCB and connect to the internet.
- In parallel, I have been working on the machine learning. The problem we are trying to solve is a substitution cipher. Brute force was fast but noise threw it off very easily. We found a naive bayes approach and attempted to use it. It was resistant to noise but was exceedingly slow.
- Upcoming work description & expectation
- My work going forward will be focused on machine learning and battery conservation on the ESP32, now that we have a working remote listening device, we need to make sure it meets our laid out target of being able to run for 12 hours on one 200mAh battery .
- We may have to recollect the data, the collecting data by recoding individual key presses may not have been the best idea. Preliminary tests using the clustering algorithm show that letters that are located physically close to each other are being put in the same cluster. This is to be expected, but we were hoping to see more separation.
- Changes to schedule description
- We are trying to reach our MVP as quickly as possible, we are seeing routes for optimization for the PCB as well as our processing pipeline, we are making a record of them but right now our main focus is on achieving the correctness. These optimizations, especially for the processing pipeline will come in handy when we try to reduce our compute time.
- We have fallen behind in the machine learning, we will be collectively working on it starting next week.
Team:
Accomplishment #1 description & result
- We built the second revision of the PCB, initially it seemed to draw too much voltage and was having the same heating problem as last time. We found out that it was because we left one of the pins on the buck-boost converter hanging that we should have tied to the output. We managed to fix this with a botch wire.
- We also managed to program the board and run our program on it. Next week we will be testing the microphone and making sure the peripherals work as intended.
- James managed to extract cepstral and fft features. He ran K-means clustering on it, but it yielded poor separation on keys that are physically close to each other on the keyboard.
Upcoming work #1 description & expectation
- After spring break, we need to try and get our new pcb working. It should work now that we use a buck boost converter.
- We need to extract features so that we can begin the machine learning. We will be using cepstral, fft and TDoA to cluster the features.
- We also hope that TDoA will serve as a highly distinguishing feature. Now that we have multiple working sensor packages (both breadboarded and PCB), we can begin to incorporate TDoA data into the clustering.
Changes to schedule description
- There will be a major shift from embedded coding and PCB design to machine learning and signal processing. We need to achieve our MVP as soon as possible so that we can begin refinement and testing.
Any other major change to your project on a high level
- There are no major changes to the project at this moment.
Team:
Accomplishment #1 description & result
- We built our first revision of our PCB, Upon testing the PCB, we discovered the ESP32 was getting very hot, much hotter than it should be in normal operation. The issue was that 5V was being put into the 3.3V pin on the ESP32. This required switching from a boost converter to a Buck-Boost converter to step the voltage both up and down. A new schematic has been created and the order will be put out soon.
- James and Ronit collected labeled data for every single key.
- James and Ronit implemented the keystroke separation as described by the paper by Zhung et al. After some noise reduction, we were able to get the keys separated rather well.
Upcoming work #1 description & expectation
- After spring break, we need to try and get our new pcb working. It should work now that we use a buck boost converter.
- We need to extract features so that we can begin the machine learning. We will be using cepstral, fft and TDoA to cluster the features.
Changes to schedule description
- We will try to get the machine learning as soon as possible, as we think it will be the most challenging portion of our project.
Any other major change to your project on a high level
- There are no major changes to the project at this moment.