Our largest major risk as of right now is determining how much we can do on an FPGA. More specifically, we are trying to experiment on whether we can do human pose estimation using an FPGA, as we believe that it could make a strong impact in boosting our performance metrics. We do have a contingency plan of doing HPE and our RNN on an Nvidia Jetson if we are unable to get this working though. That said, to best manage this risk, we are trying to frontload as much of that experimentation as possible so that if we do have to pivot, we can pivot early and stay relatively on schedule. Our other risks our echoes of the ones of last week. We have looked into the latency issues, mainly concerning the bottleneck of human pose estimation. Based on research into past projects using AMD’s Kris KV260, we do believe that we should be able to manage our latency so it isn’t a bottleneck.
As per last week, our major change is related to our risk above, in that, we want to try and split the HPE model and our RNN so that the HPE model is on the FPGA and the RNN is on the Jetson. After experimentation, we can find that we can just pull the vector data of each landmark from Mediapipe’s hand pose estimation model, allowing us to pass that into an RNN rather than an entire photo. The main reason we made this pivot is that we believe that we can have a smaller model on the Jetson in comparison to the CNN-RNN design we originally planned. This gives a bit more room to scale this model to adjust for per-word accuracy and inference speed. Luckily, as we have not begun extensive work on developing the RNN model, there is not a large cost of change here either. In the case that we are not able to have the HPE model on the FPGA, we would have to be a bit more restrictive on the RNN model size to manage space, but we would still most likely keep the HPE-to-RNN pipeline.
There has not yet been any update to our schedule as of yet, as a lot of our pivots does not have a large impact on our scheduling.
Below, we have some images that we used for testing the hand pose estimation model that Mediapipe offers:
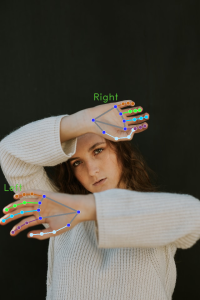
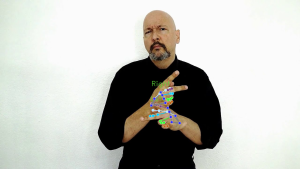

Impact Statement
While our real-time ASL translation technology is not focused specifically on physical health applications, it has the potential to profoundly impact mental health and accessibility for the deaf and hard of hearing community. By enabling effective communication in popular digital environments like video calls, live-streams, and conferences, our system promotes fuller social inclusion and participation for signing individuals. Lack of accessibility in these everyday virtual spaces can lead to marginalization and isolation. Our project aims to break down these barriers. Widespread adoption of our project could reduce deaf social isolation and loneliness stemming from communication obstacles. Enhanced access to information and public events also leads to stronger community ties, a sense of empowerment, and overall improved wellbeing and quality of life. Mainstreaming ASL translation signifies a societal commitment to equal opportunity – that all individuals can engage fully in the digital world regardless of ability or disability. The mental health benefits of digital inclusion and accessibility are multifaceted, from reducing anxiety and depression to fostering a sense of identity and self-worth. [Part A written by Neeraj]
While many Americans use ASL as their primary language, there are still many social barriers preventing deaf and hard of hearing (HoH) individuals from being able to fully engage in a variety of environments without a hearing translator. Our product seeks to break down this communication barrier in virtual spaces, allowing deaf/HoH individuals to fully participate in virtual spaces with non-ASL speakers. We’ve seen a drastic increase in accessibility to physical meeting spaces due to the pandemic making platforms like Zoom and Google Meet nearly ubiquitous in many social and professional environments. As a result, those who are immunocomprimized or have difficulties getting to a physical location now have access to collaborative meetings in ways that weren’t previously available. However, this accessibility hasn’t extended to allow ASL speakers to connect with those who dont know ASL. Since these platforms already offer audio and visual data streams, integration of automatic closed captioning has already been done on some of them, like Zoom. The development of models like ours would allow these platforms to further their range of users.
This project’s scope is limited to ASL to prevent unecessary complexity since there are over 100 different sign languages globally. As a result, the social imapact of our product would only extend to places where ASL is commonly used. ASL is commonly used in the United States, Philippines, Puerto Rico, Dominican Republic, Canada, Mexico, much of West Africa and parts of Southeast Asia. [Part B written by Sandra]
Although our product does not have direct implications on economic factors, it can affect the economic factors in many indirect ways. The first method is by improving the working efficiency of a large subgroup of the population. Currently, the deaf and hard-of-hearing community have a lot of hurdles when working on any type of project because the modern work environment is highly reliant on video conferencing. By adopting our product, they will become more independent and thus will be able to work more effectively, which will in-turn improve the output received by their projects. Overall, by improving the efficiency of a large subgroup of the population, we would effectively be improving the production of many projects and thus boosting the economy. Additionally, our entire project is built using open source work and anything we build ourselves will also be open source. By adding to the open source community, we are indirectly boosting the economy because we are making further developments in this area much easier. We are only targeting ASL and there are many other sign languages which will eventually have to be added to the model. Thus, further expansion on this idea becomes economically friendly (due to reduction in production time and cost savings) and leads to more innovation. [Part C written by Kavish]