This week I spent the majority of my time working on the function “locate_drum_rings” which is triggered via the webapp and initiates the process to find the (x,y) location of the drum rings as well as their radii. This involved developing test images/videos (.mp3), implementing the actual function itself, choosing to use scikit-image over cv2’s hough_circles, tuning the parameters to ensure the correct circles are selected, and testing the function in tandem with the webapp. In addition to implementing and testing this function, I made a few more minor improvements to the other endpoint on our local server “receive_drum_config” which has an error in its logic regarding saving received sound files to the ‘sounds’ directory. Finally, I changed how the central controller I described in my last status report worked a bit to accomodate 2 drumsticks in independent threads. I’ll explain each of these topics in more detail below:
Implementing the “locate_drum_rings” function.
This function is used at the start over every session, or when the user wants to change the layout of their drum set in order to detect, scale, and store the x.y locations and radii of each of the 4 drum rings. It is triggered by the “locate_drum_rings” endpoint on the local server when it receives a signal from the webapp s follows:
from cv_module import locate_drum_rings
@app.route(‘/locate-drum-rings’, methods=[‘POST’])
def locate_drum_rings():
# Call the function to detect the drum rings here
print(“Trigger received. Starting location detection process.”)
locate_drum_rings()
return jsonify({‘message’: ‘Trigger received.’}), 200
When locate_drum_rings() is called here it starts the process of finding the centers and radii of each of the 4 rings in the first frame of the video feed. For testing purposed I generated a sample video with 4 rings as follows:
1.) In MATLAB, I drew 4 rings with the radii of actual rings we plan on using (8.89, 7.62, 10.16, 11.43) at 4 different, non-overlapping locations.
2.) I then took this image and created a 6 second mp4 video clip of the image to simulate what the camera feed would look like in practice.
Then during testing, where I flag testing=True to the function, the code references the video as opposed to the default webcam. One pretty significant change however was that I decided not to use CV2’s Hough circles algorithm and instead use scikit-image’s Hough circles algorithm predominantly because it is much easier to narrow down the number of detected rings to 4, where with CV2’s it became very difficult to do so accurately and with varying radii (which will be encountered due to varying camera heights). The function itself opens the video and selects the first frame as this is all is needed to determine the locations of the drum rings. It then masks the frame and identifies all circles it sees as present (it typically detects circles that aren’t actually there too, hence the need for tuning). Then I use the “hough_circle_peaks” function to specify that it should only retrieve the 4 circles that had the strongest results. Additionally, I specify a minimum distance between 2 detected circles in the filtering process which serves 2 purposes:
1.) to ensure that duplicate circles aren’t detected.
2.) To prevent the algorithm from detecting an 2 circles per ring; 1 for the inner and 1 for the outer radius of the rubber rings
Once these 4 circles are found, I then scale them based on the ratios outlined in the design report to add the equivalent of a 30mm margin around each ring. For testing purposes I then draw the detected rings and scaled rings back on the frame and display the image. The results are shown below:
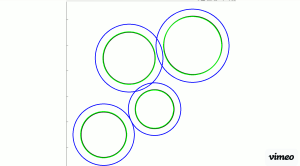
The process involved tuning both values for minimum/maximum radii, minimum distances between detected circles, and the sigma value for the edge detection sensitivity. The results of the function are stored in a shared variable “detectedRings” which is of the form:
{1: {‘x’: 980.0, ‘y’: 687.0, ‘r’: 166.8}, 2: {‘x’: 658.0, ‘y’: 848.0, ‘r’: 194.18}, 3: {‘x’: 819.0, ‘y’: 365.0, ‘r’: 214.14}, 4: {‘x’: 1220.0, ‘y’: 287.0, ‘r’: 231.84}}
Where the index represents what drum the values correspond to.
Fixing the file storage in the “receive_drum_config” endpoint:
When we receive a drum set configuration, we always store the sound files in the ‘sounds’ directory under the names “drum_{i}.wav” where i is an index 1-4 (corresponding to the drums). The issue however was that when we receive a new drum configuration, we were just adding 4 more files with the same name to the directory, which is incorrect because a.) there should only ever be 4 sounds in the local directly at any given time, and b.) because this would cause confusion when trying to reference a given sound as a result of duplicate names. To resolve this, whenever we receive a new configuration I first clear all files from the sounds directory before adding the new sound files in. This was a relatively simple, but crucial fix for the functionality of the endpoint.
Updates to the central controller:
Given that the controller needs to monitor accelerometer data for 2 drum sticks independently, we need to run 2 concurrent instances of the controller module. I changed the controller.py file to do exactly this: spawn 2 threads running the controller code, each with a different color parameter of either red or green. These colors represent the colors of the tips of the drumsticks in our project and will be used to apply a mask during the object tracking/detection process during the playing session. Additionally, for testing purposes I added a variation without the threading implementation so we can run tests on one independent drumstick.
Overall, this week was successful and I stayed on track with schedule. In the coming week I plan on helping Eliot integrate his BLE code into the controller so that we can start testing with an integrated system. I also plan on working at optimizing the latency of the audio playback module more since while it’s not horrible, it could definitely be a bit better. I think utilizing some sort of mixing library may be the solution here since part of the delay we’re facing now is due to the duration of a sound limiting how fast we can play subsequent sounds.