This week I worked on multiple parts of my domain in the project, including the camera dynamics, running CUDA, and improving our YOLO model. Furthermore, I worked alongside the rest of the team in the overall integration of all the project’s subsystems.
My week started off with running our interim demo’s inference script on CUDA since I got GPU acceleration working last week. While I wished it were as simple as running the script, that of course was not the case. As a reminder, I had trouble compiling the Jetson libraries necessary for CUDA support on widely supported Python versions like 3.8, so I opted to reflash the Jetson with an image that contains the necessary libraries pre-installed, albeit at the price of sticking to an older Python version. Unsurprisingly, this led to library dependency issues as some of the libraries used in our interim demo did not support our downgraded Python. As a result, I had to also downgrade to an older version of YOLOv5, which then caused issues with importing the model as that was fine-tuned on the latest version. Long story short, I had a few painful hours of re-exporting the model and modifying the script to accommodate any differences in the new model’s behavior, but all is well that ends well as I got GPU acceleration working with YOLOv5. Compared to running inference on the CPU, CUDA inference is unsurprisingly smoother, which should aid us in meeting our inference time use case requirement.
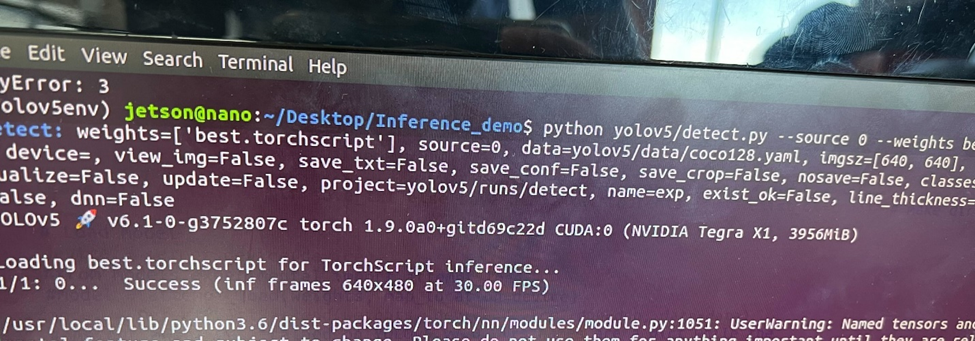
With GPU acceleration working, I then moved on to configuring the Oak-D SR camera. I learned the hard way that a high bandwidth USB 3.0 to USB type C cable is necessary to properly interface with the camera. I followed the company’s documentation for setting up the camera and running their demo Python scripts, which had variable success from the get-go as only the grayscale ones would work. After some tinkering I found out that initializing the SR’s RGB camera’s was different than the remaining models (or at least I assume), so I adapted the new initialization steps and got the demos working in color that way. I mainly tinkered with the camera control’s demo, which places you in a sandbox setting where you could fine-tune the many settings of the camera to your liking. Additionally, I tried implementing a quick script for depth detection as that would be helpful for detecting nearby objects on the belt, but with variable success. Note that all the aforementioned was done on my laptop.
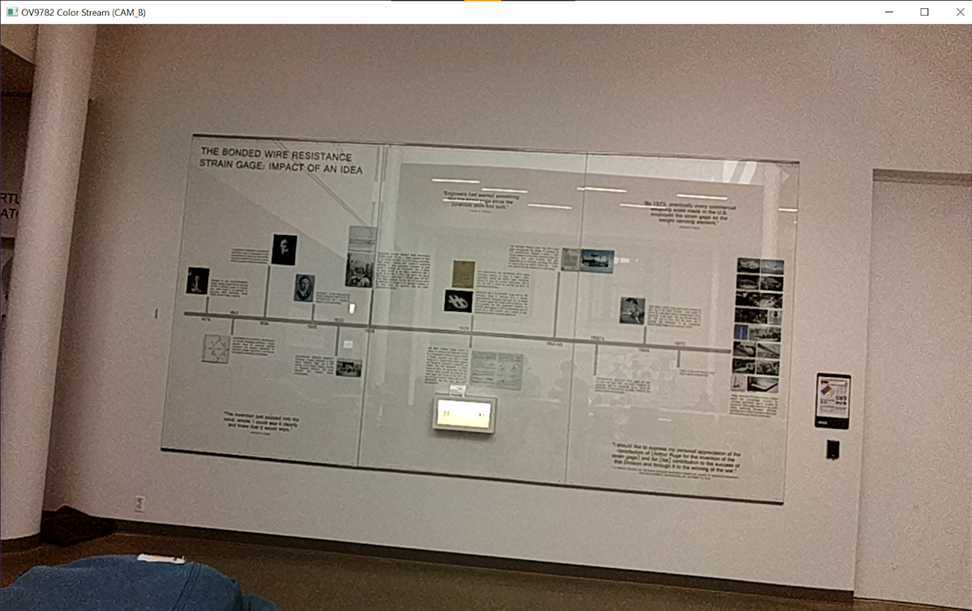
Unfortunately, getting the camera to work on the Jetson was not a cake walk, primarily because its power and memory usage caused the Jetson to outright shutdown due to stress. Initially I had the camera function as a generic webcam using a UVC (USB Video Class) Python script before connecting to it using our interim demo script. To place less strain on the Jetson, I switched to handling everything in one script in addition to adding further optimizations like installing a less memory intensive desktop interface, and optimizing my model’s weights to short floats (from floats). The camera feed was stable since, although slightly choppy. The bummer is despite all I have done to get the Oak-D working, our model’s performance with it was inferior to the generic webcam, likely due to color differences. I tried fine-tuning the camera output as much as possible to optimize our accuracy to what it was before but with variable success. So we decided to stick with the generic webcam used for the interim demo. I optimized the camera’s feed to reduce the glare on object’s, an issue that made certain objects undetectable, using the Video4Linux2 interface. We hope to revisit using the Oak-D SR in addition to its depth mapping if time permits.
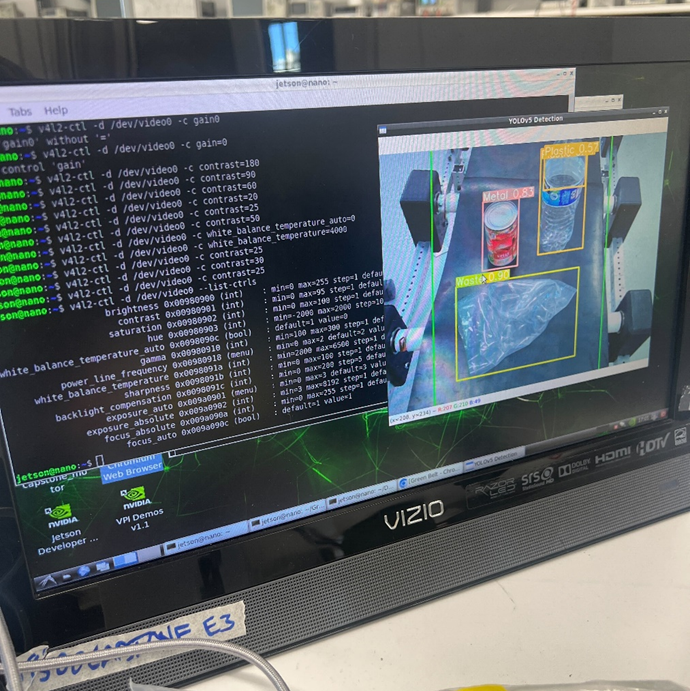
My final main contribution was switching to a different YOLOv5 model, as recommended by John. John found a paper tackling a similar problem, which provided a much larger dataset (almost 10,000 samples compared to the 1500 of the TACO dataset) as a part of its methodology. While there were also model files included, the documentation was incomplete in addition to the fact the model’s parameters were not labeled in English, which discouraged using them directly. I tinkered with some settings and trained a YOLOv5s model from scratch on the new dataset, which seems to be an improvement thus far. Note that I had to adjust our main inference script to accommodate the different waste categories of the new model. The new categories (food waste, textile, wood, leather, and ceramic) are all treated as trash for our project’s scope. Nevertheless, I do not anticipate the model will detect said classes as it is unlikely we will supply them during our testing.
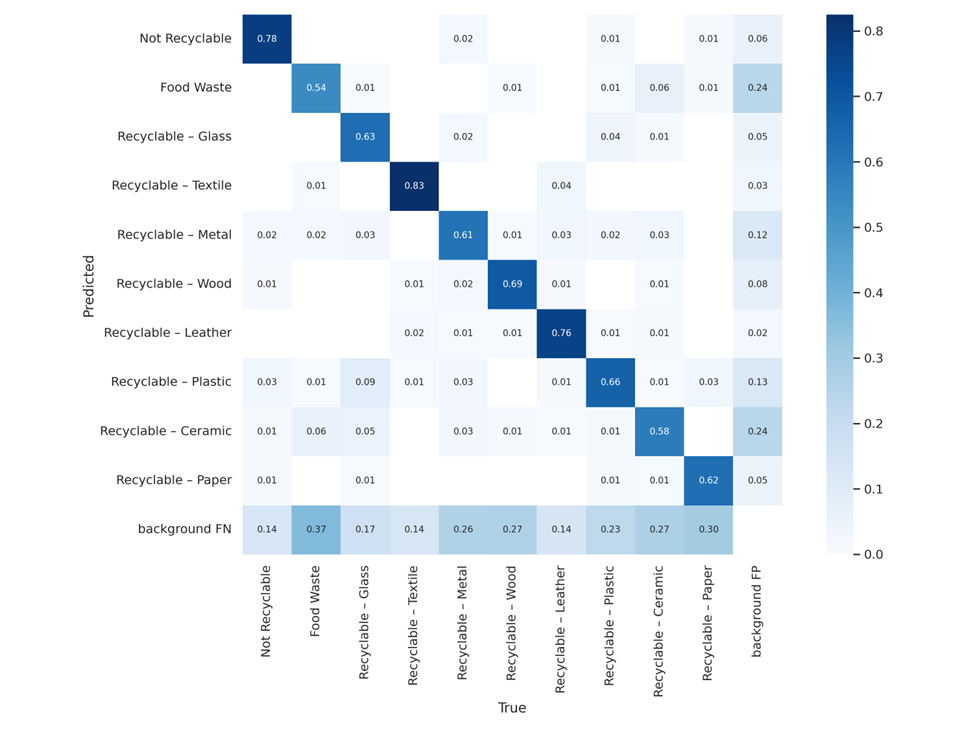
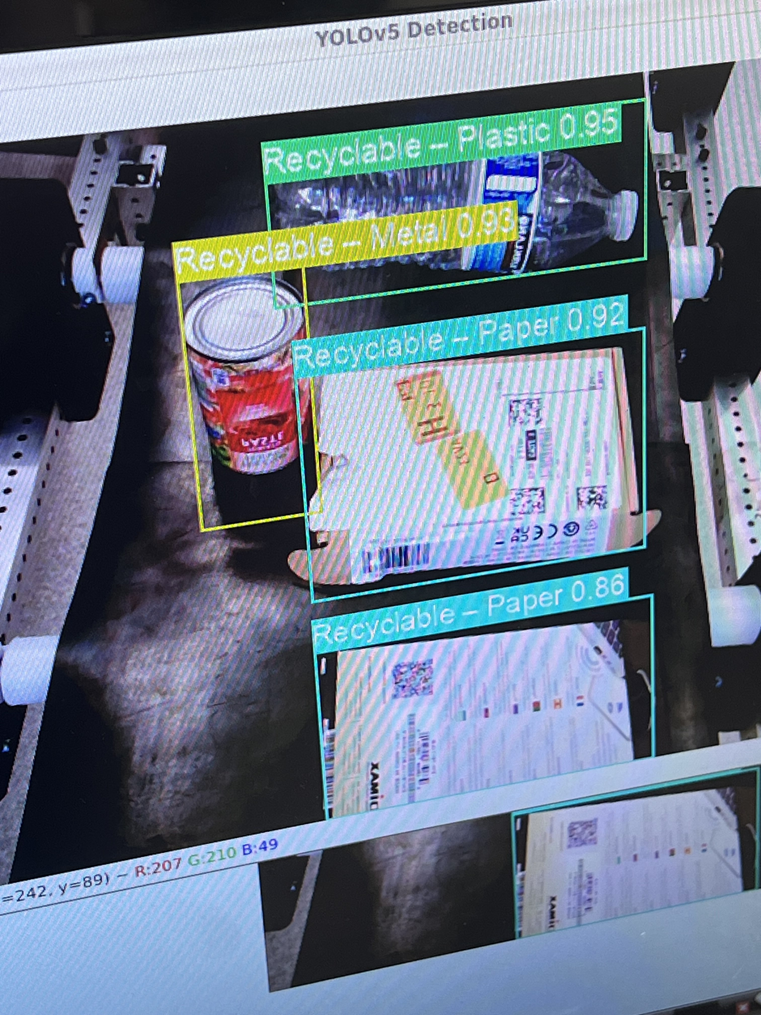
Finally, I helped Erin with fixing our motor speed issues in addition to interfacing between the YOLO script and UI plus actuators.
Additional Question
I did have to learn many things on the fly, such as fine-tuning and training a YOLO model, setting up a Jetson with CUDA from scratch, in addition to using a non-plug and play high-end camera. To be brutally honest, a lot of that learning has been facilitated by generative AI. For example, while I have used YOLO before for another project, ChatGPT helped guide me more depth through the different scripts of YOLOv5 in addition to resolving some bugs here and there. Furthermore as a Windows user, having a co-pilot for navigating certain Linux features (e.g. v4l2) has been a treat. Other than that, I did watch videos as well as read papers forum posts to learn other things, such as interfacing with the Oak-D cameras and getting RGB to work on the SR. Reading research papers was especially helpful in setting up and improving our object detection model.