What I accomplished this week:
This week, I made significant progress on both the physical and software components of the project, dedicating a lot of time to work on the design, fabrication, coding, and electronic components of SmartWatt.
-
Mechanical Design & Fabrication:
I completed a full redesign of the house structure in CAD to incorporate finger joints, which greatly improved the stability and robustness of the model. This change ensures the structure is more durable and can handle repeated assembly and disassembly without loosening or falling apart.
After finalizing the design, I finished laser-cutting all of the components for the house using both wood and acrylic materials. This marks a major milestone in the physical build of the prototype.
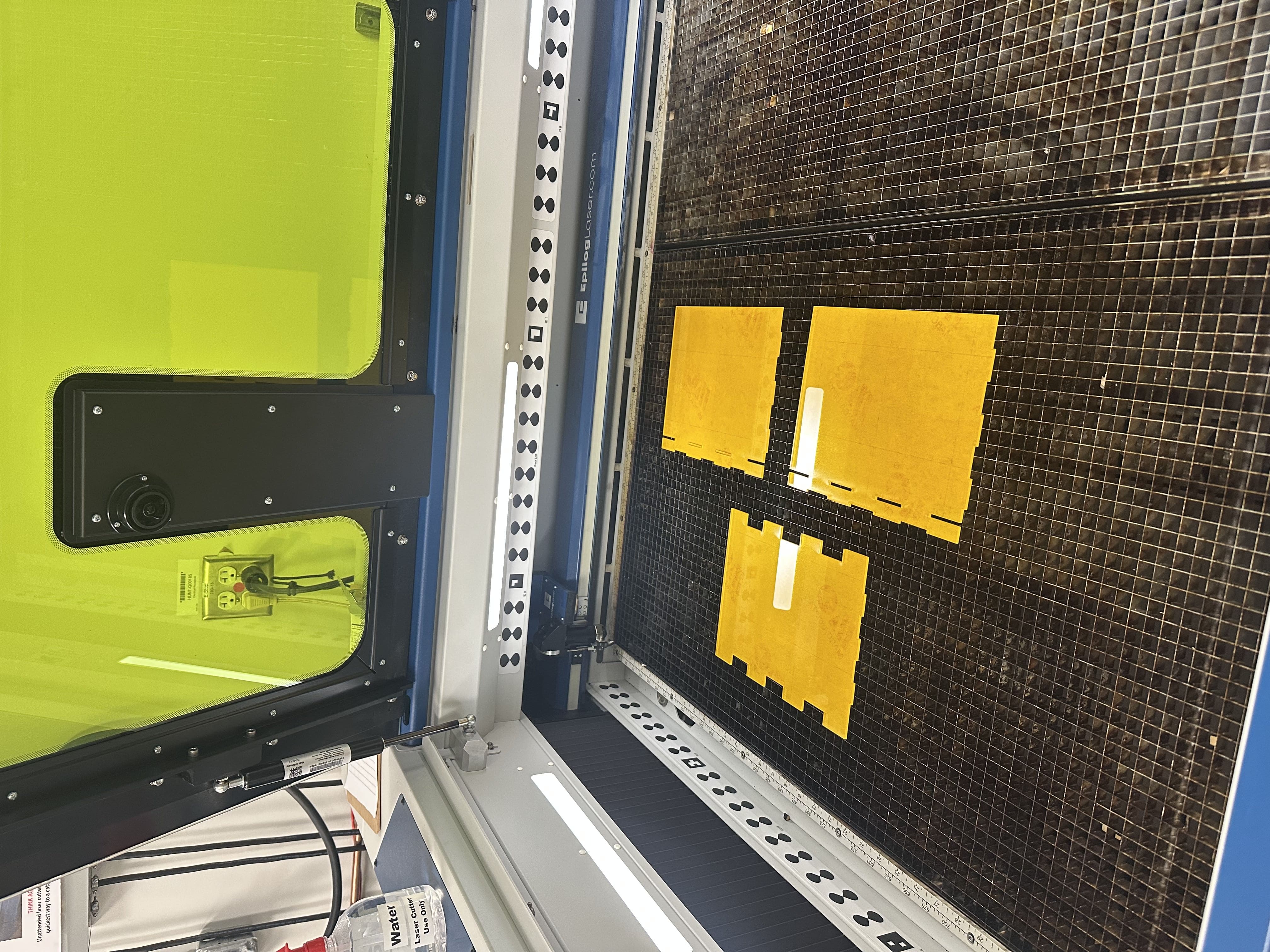
-
Software & Electronics Development:
I coded the chatbot system that will serve as one of the primary interfaces for user interaction. After evaluating different models, I decided to use an OpenAI LLM to power the chatbot due to its flexibility, customization potential, and strong performance in conversational tasks.
On the hardware side, I successfully set up and programmed an LED ring to visualize the passage of time, where one minute equals one simulated day. This ring offers an intuitive way to represent time-based changes in the home’s energy behavior.
.
Unfortunately, I am not able to upload a video demo because of the file size limits.
Project schedule status:
My progress is currently on schedule. With major fabrication and basic chatbot functionality complete, we are ready to move into final integration in the final week.
Goals for next week:
-
Feed custom user data into the OpenAI LLM so the chatbot can give energy-saving recommendations tailored to a specific user’s home and help the user make sense of their dashboard
-
Begin integration between the TypeScript frontend and the Python backend, ensuring smooth communication between the user interface and the underlying AI and hardware logic.
New tools and knowledge acquired:
-
I learned how to use CAD to design a house, including how to sketch, add dimensions, and combine parts into a full assembly using mates. To do this, I watched a series of Fusion 360 YouTube tutorials and experimented by prototyping small sections before scaling up to the full house model.
- I also learned how to laser cut. I went to Ideate’s makerspace in Hunt Library and was taught by a friendly laser cutting attendant who walked me through the steps of setting up the machine and preparing my design for the cut.
- For the chatbot, I familiarized myself with the OpenAI API, especially prompt engineering and how to structure memory inputs. I referenced the OpenAI documentation and read through a couple of online tutorials.
These informal learning strategies—particularly hands-on experimentation and community support—were essential in rapidly acquiring the skills needed.