🟢 Progress This Week
Central Server Architecture Refinement
- Shifted OCR processing from central server to onboard dash cam after faculty & TA feedback.
- Refined layered architecture with API Gateway, Lambda, S3, and DynamoDB for scalability.
- Updated central server design to reflect this change.
- Before: OCR was centralized.
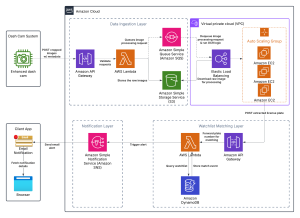
- After: ALPR & OCR runs on the dash cam; the central server focuses on match verification & notifications.
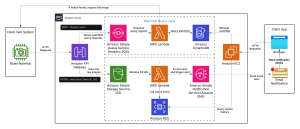
API Design & System Components
- Defined RESTful resource structure for plates, matches, and notifications and esigned API endpoints for central server:
- Detection upload
- Match storage & retrieval
- Police portal access
- Researched AWS-based load testing approaches for handling 314K concurrent requests.
Web Application Enhancements
- Drafted design document outlining:
- Vision & Key Features: Watchlist management, real-time alerts, match history search.
- Use Cases: Detailed core flows for authentication, watchlist updates, and search.
- Database Considerations: Decided on DynamoDB (watchlists) & RDS (match history).
- Defined the interactions between web app backend and central backend.
Presentation Preparation
- Began preparing slides for the upcoming design review presentation.
- Drafted content covering architecture, security, scalability, and use case breakdown.
🔜 Next Steps
-
Design Report 🫠
- API Gateway Setup & Testing
- Configure routes for core API endpoints.
- Manual testing to make sure API Gateway is properly set up.
- Database Schema Design & Setup
- Implement schema for DynamoDB (watchlist storage) and RDS (match history).
- Ensure indexing and query optimization.
- Lambda Integration for Message Queueing
- Implement Lambda function to process incoming detections asynchronously.
⚠️ Challenges & Open Questions
Legal Compliance & Scope Definition
We researched legal considerations but need to define the project scope—we cannot implement everything.
- What we will support:
- Controlled officer access with authentication & audit logs.
- Watchlist justification (officers must provide a case number to track plates).
- Data retention policy (match records auto-expire after 90 days).
- What is out of scope:
- Full identity verification system for officers.
- Integration with external law enforcement databases.
- System-wide hacking/data abuse protections (beyond standard security measures).
Privacy & Data Security
- Encrypt all stored & transmitted data (end-to-end encryption).
- Require officers to justify searches (case number, reason) to prevent misuse.
📌 Overall Status
✅ Currently on track.
The project has been demanding, especially since we aim to design a distributed, scalable system that can efficiently handle high loads. However, we have made a lot of progress on the design side, and we feel confident to start the implementation phase.