This week, I worked on setting up the Arducam wide-lens camera on the Jetson, setting up the keypad to work on the Raspberry Pi, setting up the haptics sensors to work on the Raspberry Pi, and setting up socket communication under the same wifi subnet between the Raspberry Pi and my laptop. The first task I did was setting up the Arducam wide-lens camera by installing the camera drivers on the Jetson. The process was quite smooth compared to the OV2311 Stereo Camera since this wide-lens camera relied on the USB protocol which is a lot more robust.
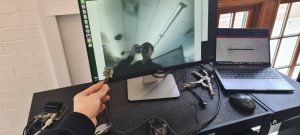
From the image you can see the quality is decent and the camera has a 102 FOV.
Next I worked on communication between the Raspberry Pi and the laptop using sockets. I set up a virtual environment on the Raspberry Pi and installed all the necessary dependencies to set this up. After this I set up the keypad on the Raspberry Pi using GPIO pins. Once the Raspberry Pi detects that a key is pressed, it sends data to the laptop which runs the A* path planning module and has the occupancy matrix visualized. The keypad is able to select different destinations on the occupancy matrix via network connection.
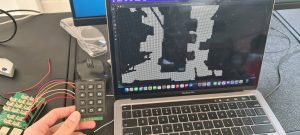
Once the laptop runs path-planning and determines the next move for the user, it sends the directions back to the user (Raspberry Pi) through the same socket connection. I then set up the haptic vibration motors which is also on the Raspberry Pi. I had to use different GPIO pins to power the vibration motors since some pins were already used for the keypad.
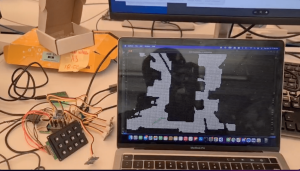
The Python code on the Raspberry Pi was able to detect which direction the user had to move in next and vibrate the corresponding haptic vibration motors. Our haptic vibration motors had slightly short wires, so we might need an extension for the final belt design.
I believe my progress is on schedule. Currently, the next main milestone for the team is to integrate the UWB sensor localization with the existing occupancy matrix. This is our last moving part in terms of project functionality. The last milestone for this project would be to integrate the Arducam wide-lens camera instead of the phone camera.
Next week, I hope to work with Kevin on integrating the UWB sensor localization with the occupancy matrix generated from the segmentation model and down-sampling. Currently, the user location is simulated through mouse presses, but we want it to be updated on our program through data received from the UWB tags. We would have to think about the scale factor of the camera frame and how much it has been downsampled, and apply the same scale to the UWB reference frame.
To verify my components of the subsystem, I would first test the downsampling algorithm and the A* path-planning module on different environments. I would feed the pipeline around 10-15 bird’s eye view images of indoor spaces. After the segmentation model, I would note if the downsampling does a good job of retaining the required resolution and identifying obstacles and free space. I would empirically measure the occupancy matrix on whether 95% of obstacles are actually still labeled as obstacles. To meet our use case requirement of not bumping into obstacles, I would come up with a metric that shoots for over-detection of obstacles rather than under-detection. Our current downsampling algorithm creates a halo effect around the obstacles, which would meet this requirement. For the communication requirements between the Raspberry Pi (the user’s belt) and the laptop, there is already minimal processing time. The A* path planning algorithm also has minimal processing time, so the user will have real-time updates on which direction to go through haptic sensors that meets our timing requirements. For percentage of navigation to the right location, I would check whether the haptic sensors always vibrate in the right direction out of 10 tries. If there is some calibration required by a compass, that would be taken into account as well.