This week, I first tried to find an alternate to the OV2311 Stereo Camera that was not working with the Jetson. The camera had some issues and so we decided to use a phone camera that would be sent to the laptop for processing. We are going to move forward with this solution for the time being so that we can get to MVP and then decide to tweak some of the components later. I was able to capture images from my phone camera’s wide lens view and receive it on my laptop using Python’s OpenCV library. The frame of the indoor environment being stored as an OpenCV video capture is perfect for us to process later.
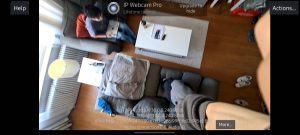
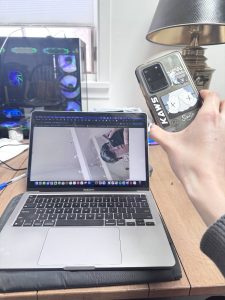
After this, I tried to experiment with some CV segmentation models that could classify the environment into either obstacles or free space. The models I tried were Segment Anything Model (SAM), Mask2Former Universal Segmentation, DeepLabV3 with COCO weights, and DeepLabV3 with Pascal weights. These models are pre-trained with mostly general data, and so they did not work as well on indoor environments from a top-down bird’s eye view as seen in the following images.
SAM Model With Hamerschlag Hall View:
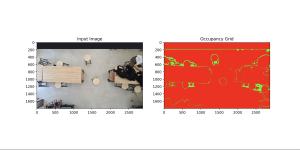
SAM Model + Mask2Former Universal Segmentation:
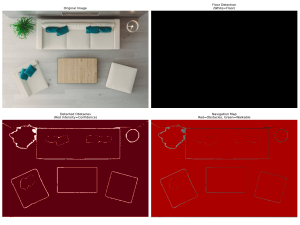
DeepLabV3 Model with COCO Weights:
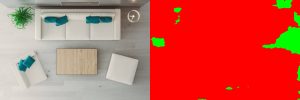
DeepLabV3 Model with Pascal Weights:
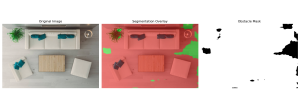
As seen from these images, the closest model that is able to at least outline the edges of the furniture is the SAM Model. Charles was able to tune the model such that it fills in the entire furniture and so we were able to get a working model from that. Thus, we are now going to base our segmentation model on the modified SAM Model.
Next, I started working on the D* Lite algorithm. I was able to create a UI that displays the person and the target in a 2D occupancy matrix (one that is similar to the one generated from the modified SAM model). The 2D occupancy matrix contains 0 for free space and -1 for occupied space. The D* algorithm recalculates the shortest path starting from the target to the person and uses heuristics to speed up the algorithm. Currently, I am controlling the person using arrow keys but these will be replaced with UWB sensors after we get that working. The person will also be navigated in the direction of the lowest cost.
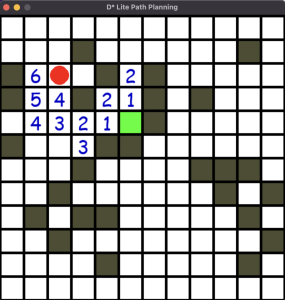
My progress is slightly behind schedule as we were not able to get the OV2311 camera working. However, we have decided to use the phone camera as an alternative so we can move forward with our project. Once we have a working model for all the parts, we can consider switching back to the stereo camera instead of a phone camera. With the phone camera working, we are able to make significant progress on the software end of our project this week, so we expect similar progress going forward.
Next week, I hope to integrate the phone camera, SAM CV Model, and D* Lite Path Planning algorithm all into the same pipeline. Currently, these are all working as lone components, but we have to ensure that the output of one stack can feed into the input of the other. Once I am able to integrate all these components, our software stack will be mostly done and we could plug in hardware components as we move forward.