The most significant risks that could jeopardize the success of the project are the PID controller not working and various filtering issues. Currently we are running into issues with the PID not able to get the drone to hover exactly level, instead it is drifting off to the side. We are managing these risks by working on the drone itself as diligently as possible to showcase the controller working to some degree. We have enough to showcase at the final show, but our contingency plan is to make the drone manually controlled.
The major change that we have made to this system is using a manually controlled drone to showcase the PID controller. This change was necessary as we cannot immediately get the lawnmower operation working even though we have the algorithm ready. Our new schedule is having everything done by Wednesday.
Unit tests for CV:
* Yolov8n is able to get camera feed
* Yolov8n is able to detect any object (can be wrong)
* Yolov8n is able to detect any object correctly
* Yolov8n is able to detect object we want to detect
* Yolov8n is able to detect object image coordinates correctly
Unit Tests for Tracking:
* Tracking is able to receive image input
* Tracking is able to receive image coordinate for bbox
* Tracking is able to parse the inputs into ints
* Tracking is able to ouptut the distance vectors from the object detect
* Tracking is able to compute the direction
Unit Test for Radio:
* Radio is able to receive bytes
* Radio is able to send bytes
* Radio range testing
Unit Tests for Drone IMU:
* Drone IMU calibaration
* Drone IMU Kalaman filter outputs correct values
* Drone IMU Kalaman filter with IMU reading cause no drift
* Drone IMU maintains correct readins after vigorus movements
* Drone controlls operating at 200+Hz
Unit Tests for Drone PID:
* Drone PID controller is able to get set point error
* Drone PID controller is able to utilize kalman filter data to calculate D
* Drone PID controller is able to calculate error required for I value
* Drone PID controller shows the drone trying to compensent with only P value
* Drone PID controller shows drone’s leveling gets dampned with addition of D value
* Drone PID controller shows I value is able to take care of steady state error


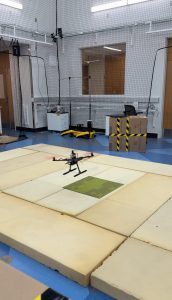